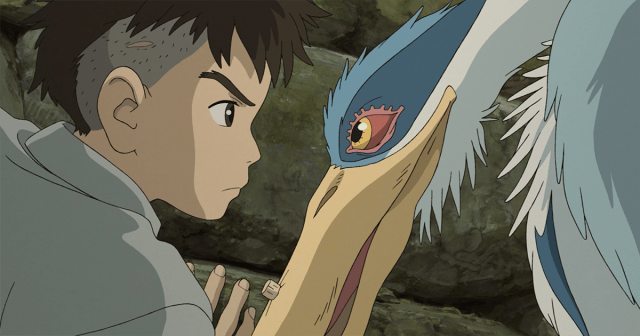
TL;DR
- Generative AI tools like Midjourney and DALL-E 3 can unintentionally produce copyrighted content, challenging the originality and legal boundaries in media.
- The use of copyrighted material in AI training raises significant ethical and legal concerns, with potential risks for creators and developers across the media industry.
- Solutions include retraining AI models with licensed data and implementing filters for problematic queries, but these pose practical challenges in implementation and effectiveness.
- AI Researchers Gary Marcus and Reid Southen emphasize the need for creators, developers, and stakeholders to collaboratively address these challenges, advocating for transparency, ethical development, and respect for intellectual property.
READ MORE: Generative AI Has a Visual Plagiarism Problem (IEEE Spectrum)
Imagine a world where iconic images from your favorite movies are replicated, not by fans, but by AI systems like Midjourney and DALL-E 3 — all without any direct instruction.
Generative AI tools like Midjourney and DALL-E 3 are capable of creating images that, while not directly instructed to do so, can be strikingly similar to well-known characters and scenes from popular media. This capability, though technologically impressive, poses a risk of infringing on intellectual property rights, inadvertently creating a conflict between AI innovation and copyright law.
This phenomenon, explored in depth by Gary Marcus and Reid Southen in IEEE Spectrum, opens a Pandora’s box of ethical dilemmas and legal quandaries, challenging the very notion of originality.
The issue came to head in early January, when Midjourney was accused of compiling a database of thousands of artists, including prominent names like Hayao Miyazaki, Matt Groening, and Keith Haring to train its text-to-image tool.
The database, which included various artistic styles and names, was allegedly used to mimic these artists’ styles, raising issues of copyright infringement and the ethical use of artists’ work in AI training. The revelations arrived amid an ongoing class-action lawsuit between a group of artists and Stability AI, Midjourney and DeviantArt, as It’s Nice That’s Liz Gorny reports:
“A leaked Google Sheet was circulating online on 31 December 2023 featuring visual styles like ‘xmaspunk,’ ‘carpetpunk’ and ‘polaroidcore’ and thousands of individual artist names. The list was allegedly put together by developers at Midjourney; screenshots shared by Riot Games’ Jon Lam (founder of advocacy moment Create Don’t Scrape) appeared to show developers discussing sourcing artists and styles from Wikiart and laundering datasets to avoid copyright infringement.”
READ MORE: Hayao Miyazaki, Matt Groening and Keith Haring among artists allegedly used to train Midjourney AI (It’s Nice That)
The Plagiarism Challenge in Generative AI
Marcus and Southen detail how these advanced AI models can unintentionally produce content that closely resembles copyrighted material, providing concrete examples to illustrate the issue. “In each sample, we present a prompt and an output,” they write. “In each image, the system has generated clearly recognizable characters (the Mandalorian, Darth Vader, Luke Skywalker, and more) that we assume are both copyrighted and trademarked; in no case were the source films or specific characters directly evoked by name.”
Such instances of visual plagiarism are not deliberate actions by these AI models but are instead a byproduct of their training and operational mechanisms. Marcus and Southen explain that large neural networks, which form the backbone of these AI tools, “break information into many tiny distributed pieces; reconstructing provenance is known to be extremely difficult.” This fragmentation means that an AI tool trained on copyrighted material cannot discern between copyrighted and non-copyrighted inputs during its learning process, leading to outputs that inadvertently mirror existing copyrighted works.
This technical backdrop poses a complex challenge. On one hand, the ability of AI to generate such detailed and nuanced content is a testament to its technological advancement. On the other, it brings to light the imperative need for mechanisms that can distinguish between creative inspiration and copyright infringement.
Ethical and Legal Implications
The inadvertent production of copyrighted material by generative AI tools like Midjourney and DALL-E leads to a host of ethical and legal issues, as Marcus and Southen point out: “The very existence of potentially infringing outputs is evidence of another problem: the nonconsensual use of copyrighted human work to train machines.”
The potential legal ramifications are significant. The duo notes that “no current service offers to deconstruct the relations between the outputs and specific training examples,” meaning that it is challenging to trace the origins of AI-generated content back to specific inputs. This opacity in AI’s functioning can lead to legal complexities for creators who might unknowingly use AI-generated content that infringes on existing copyrights.
Marcus and Southen take a strong position on the issue, calling for a moral reckoning in the way AI technologies are developed and utilized, particularly in fields reliant on creative content. “In keeping with the intent of international law protecting both intellectual property and human rights, no creator’s work should ever be used for commercial training without consent,” they state.
Potential Solutions and Their Implications
In addressing the challenges posed by AI-generated visual plagiarism, Marcus and Southen propose several potential solutions, each with its own implications. Their suggestions focus on mitigating the risks of copyright infringement while maintaining the innovative momentum of AI in content creation.
One key solution offered by the authors is the retraining of AI models. “The cleanest solution would be to retrain the image-generating models without using copyrighted materials, or to restrict training to properly licensed datasets,” Marcus and Southen suggest. This approach directly addresses the root of the issue — the input data used in training AI models. However, the practicality of this solution is complex. Retraining AI models on a large scale requires significant resources and time and may impact the quality and diversity of the AI’s output, a concern for an industry that thrives on creative and diverse content.
Another proposed solution is the implementation of filters to screen out problematic queries. While this seems like a straightforward approach, its effectiveness in practice is debatable. Filtering algorithms would need to be sophisticated enough to recognize a wide range of potentially infringing content, which is a challenging task given the nuances and context-dependent nature of copyright law.
Marcus and Southen also highlight the importance of transparency and responsibility in AI development. They argue for a more ethical approach, stating, “Image-generating systems should be required to license the art used for training, just as streaming services are required to license their music and video.”
The authors acknowledge the challenges in implementing these solutions, noting that “neither [solution] is easy to implement reliably.” However, they emphasize the necessity of such measures, stating, “Unless and until someone comes up with a technical solution that will either accurately report provenance or automatically filter out the vast majority of copyright violations, the only ethical solution is for generative AI systems to limit their training to data they have properly licensed.”
The Role of Creators and Stakeholders
Creators, developers, and other stakeholders play a critical role in navigating the ethical landscape of AI, with a collective responsibility for shaping an AI environment that respects both creativity and legal boundaries, Marcus and Southen contend.
“Users should be able to expect that the software products they use will not cause them to infringe copyright,” they say, emphasizing the duty of developers to create AI tools that not only foster innovation but also safeguard against unintentional legal infringements.
The authors argue for a concerted effort to include artists and creators in the conversation about AI development, suggesting that their insights can lead to more ethically sound and legally compliant AI systems. This collaborative approach can help in establishing standards and guidelines that balance the innovative potential of AI with the rights and interests of creators.
Marcus and Southen also advocate for the development of software “that has a more transparent relationship with its training data,” suggesting that greater visibility into how AI models are trained can lead to more responsible use of these technologies. This transparency, they maintain, is crucial for maintaining trust among creators, users, and the public, ensuring that AI systems are used in a way that is both legally and ethically sound.
They call for a proactive stance from all parties involved in AI development and use. By embracing responsibility, collaboration, and transparency, they say, stakeholders can foster an AI environment that not only drives innovation but also upholds the core values of creativity and respect for intellectual property.
Read more of NAB Amplify’s AI content here.
Midjourney produced images that are nearly identical to shots from well-known movies and video games. Right side images: Gary Marcus and Reid Southen via Midjourney. Click here to view a larger version.
