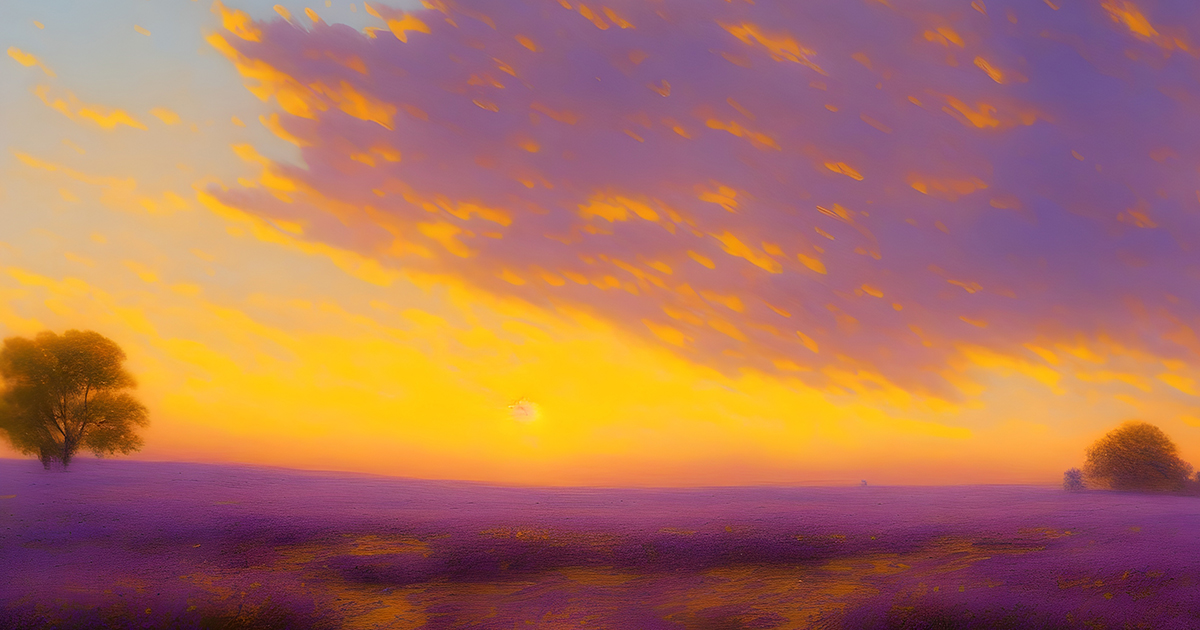
READ MORE: Visual Trends in AI Art (Amelia Winger-Bearskin)
It’s easy to say that AI art is becoming indistinguishable from human creations, but there are tell-tale signs that give the game away.
Amelia Winger-Bearskin, an artist working with AI and an Associate Professor of Artificial Intelligence and the Arts at the University of Florida, has researched and compiled a set of aesthetic conventions commonly seen in AI-generated imagery.
She breaks them down into four categories in a series of illuminating blog posts published on Medium.
Particle Systems
For as long as computer graphics have existed, particle systems have been a big part of the CG in films, data art and live performances, and “artists and designers cannot get enough of them,” Winger-Bearskin comments. Nor, it seems, can AI.
“Particle systems in game engines are beautiful,” she says, describing a style of art that perhaps attracts the Gen Z artists most likely to be working with AI today.
READ MORE: From Snapped Spiderman to Data Viz: How Particle Systems Came to Dominate Graphic Media (Amelia Winger-Bearskin)
Dada 3D
Dada 3D is the look popularized by filters to augmented images on your mobile phone. Cool 3D World, FeltZine, Vaporwave, and Instagram filters “all form part of a movement I term Dada 3D, which is something like a surrealist parlor game, a dada manifesto, and Cinema 4D got put into a blender,” Winger-Bearskin says. “This aesthetic style uses AI as mocap, detuned shaders, generative sounds, and code manipulation of game engines.”
READ MORE: How Digital Artists Explore New Frontiers of Absurdism (Amelia Winger-Bearskin)
Hyperreal
The ultra-realistic and sometimes uncanny valley rise of digital humans and deepfakes fall into the hyperreal category, which is most likely to have been created by algorithm. “I realize revenge porn is using this technique,” she caveats, “but I feel this is a foul form of harassment and not an aesthetic.”
READ MORE: Visual Trends in AI Art — Hyperreal (Amelia Winger-Bearskin)
Nightmare Corp.
Artworks in the “Nightmare Corp.” category include images created by DeepDream, Dall-E, Wombo apps, Midjourney, “and all the 1000000s of copycats we use until Dall-E is out of beta or until we can afford it.”
These images look close to something someone could make by hand, she says, but are rendered by a computer algorithm (most usually OpenAI) in 30 seconds or less. They have a unifying aesthetic in that there are smears, colors, and glitches that are still ubiquitous to each algorithm.
Winger-Bearskin delves deeper into why AI-generated images sometimes look like the stuff of nightmares.
An infamous example is the bizarre “puppy-slug” generated by Google’s DeepDream AI in 2015. A text prompt for images of dogs and the application of “dogness” to images that did not contain dogs resulted in images that are so far from puppified as to be “repulsive.” Yet DeepDream’s convolutional neural networks was trained to recognize dogs by being fed millions of pictures of dogs. So what happened?
READ MORE: Google’s Deep Dream for Dummies (Vice)
“Many people assumed that a computer’s imagination, if you could call it that, would be precise, literal, and maybe even a little bit boring,” she says. “We were not expecting to see such vivid hallucinations and organic-seeming shapes.
“The reason some of these images look so frightening is [that] these models don’t actually ‘know’ anything. These images are products of computationally advanced algorithms and calculators that can track and compare pixel values. They’re able to spot and reproduce trends from their training data, but they aren’t equipped to make sense of what they’re given.”
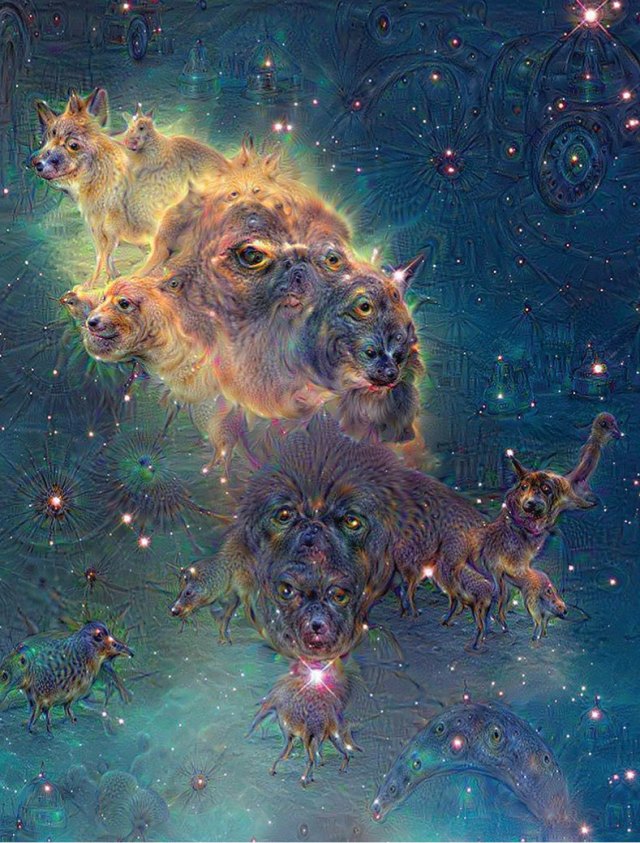
You could be forgiven for thinking otherwise, especially given the impressive results that have been generated recently with OpenAI’s Dall-E.
But when interpreting these Dall-E pieces as art, it’s helpful to keep the old Arthur C. Clarke adage in mind: “Any sufficiently advanced technology is indistinguishable from magic.”
The magic of Dall-E involves a tremendous amount of mathematics, computer science, processing power, and countless hours of work from the researchers that produced it. But the imagery produced by it and other AIs should give us a clue as to what is going on under the hood.
“A sea otter in the style of ‘Girl with a Pearl Earring’ by Johannes Vermeer.” #dalle pic.twitter.com/tjRMujvj7C
— OpenAI (@OpenAI) April 6, 2022
As Winger-Bearskin explains, Dall-E, and tools like it, work by matching words and phrases to vast stores of image data, which are then used to train generative models. The process of matching text input to the correct images requires that someone make decisions about how to sort and define the images.
The people who make these decisions are the untold millions of low-wage data entry professionals around the world, content creators optimizing images for SEO, and anyone who has ever used a Captcha to access a website. That would include you.
“Like the artisans who worked on the great cathedrals of the middle ages, these people could live and die without ever receiving credit for their work, even though the project would literally not exist without their contributions.”
She goes on to conclude that images generated in this manner are “less like paintings than they are like mirrors, reflecting our own views and values back to us, albeit through a very elaborate prism.”
For this reason, we need to be wary when we look at these pictures of the limits and prejudices contained that these models show.
READ MORE: Why AI-Generated Images Look Like the Stuff of Nightmares (Amelia Winger-Bearskin)
How Does Generative AI Work?
By Abby Spessard
READ MORE: How do DALL-E, Midjourney, Stable Diffusion, and other forms of generative AI work? (Big Think)
Generative AI is taking the tech world by storm even as the debate about AI art rages on. “Meaningful pictures are assembled from meaningless noise,” Tom Hartsfield, writing at Big Think, summarizes the current situation.
The generative model programs that power the likes of DALL-E, Midjourney and Stable Diffusion can create images almost “eerily like the work of a real person.” But do AIs truly function like a person, Hartsfield asks, and is it accurate to think of them as intelligent?
“Generative Pre-trained Transformer 3 (GPT-3) is the bleeding edge of AI technology,” he notes. Developed by OpenAI and licensed to Microsoft, GPT-3 was built to produce words. However, OpenAI adapted a version of GPT-3 to create DALL-E and DALL-E 2 through the use of diffusion modeling.
Diffusion modeling is a two-step process where AIs “ruin images, then they try to rebuild them,” as Hartsfield explains. “In the ruining sequence, each step slightly alters the image handed to it by the previous step, adding random noise in the form of scattershot meaningless pixels, then handing it off to the next step. Repeated, over and over, this causes the original image to gradually fade into static and its meaning to disappear.
“When this process is finished, the model runs it in reverse. Starting with the nearly meaningless noise, it pushes the image back through the series of sequential steps, this time attempting to reduce noise and bring back meaning.”
While the destructive part of the process is primarily mechanical, returning the image to lucidity is where training comes in. “Hundreds of billions of parameters,” including associations between images and words, are adjusted during the reverse process.
The DALL-E creators trained their model “on a giant swath of pictures, with associated meanings, culled from all over the web.” This enormous collection of data is partially why Hartsfield says DALL-E isn’t actually very much like a person at all. “Humans don’t learn or create in this way. We don’t take in sensory data of the world and then reduce it to random noise; we also don’t create new things by starting with total randomness and then de-noising it.”
Does that mean generative AI isn’t intelligent in some other way? “A better intuitive understanding of current generative model AI programs may be to think of them as extraordinarily capable idiot mimics,” Hartsfield clarifies.
As an analogy, Hartsfield compares DALL-E to an artist, “who lives his whole life in a gray, windowless room. You show him millions of landscape paintings with the names of the colors and subjects attached. Then you give him paint with color labels and ask him to match the colors and to make patterns statistically mimicking the subject labels. He makes millions of random paintings, comparing each one to a real landscape, and then alters his technique until they start to look realistic. However, he could not tell you one thing about what a real landscape is.”
Whatever your stance is on generative AI, we’ve landed in a new era, one in which computers can generate fake images and text that are extremely convincing. “While the machinations are lifeless, the result looks like something more. We’ll see whether DALL-E and other generative models evolve into something with a deeper sort of intelligence, or if they can only be the world’s greatest idiot mimics.”