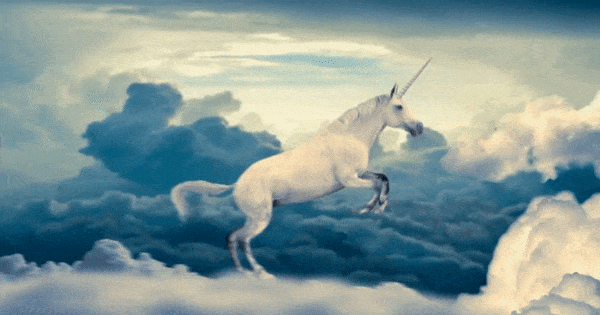
READ MORE: Understanding the Innovator’s Dilemma (Wired)
Technological change doesn’t happen overnight. It’s a long-term evolution, often decades in the making, and it’s happening right now.
Harvard Business School professor Clayton Christensen described this in his 1997 book, The Innovator’s Dilemma. It expands on the concept of disruptive technologies, a term Christensen coined in a 1995 article, “Disruptive Technologies: Catching the Wave.” The lesson that most people remember from it, as detailed by Xenios Thrasyvoulou in Wired, is that a well-run business can’t afford to switch to a new approach — one that ultimately will replace its current business model — until it is too late.
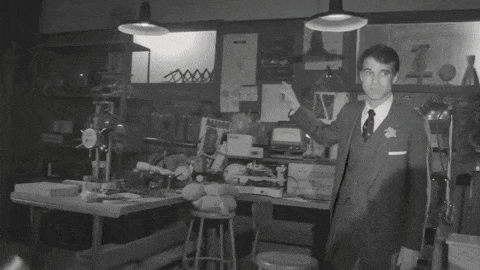
In addition, Christensen suggested that the new disruptor companies might get by for years with a far less capable technology. Some of them survive by finding a new niche they can fill that the incumbents cannot. That is where they quietly grow their capabilities.
READ MORE: Understanding the Innovator’s Dilemma (Wired)
Rodney Brooks brings this idea back in an article for IEEE Spectrum. A great example of an underperforming new approach, he writes, was the second wave of neural networks during the 1980s and ‘90s that would eventually revolutionize artificial intelligence starting around 2010.
Brooks explains, “Neural networks of various sorts had been studied as mechanisms for machine learning since the early 1950s, but they weren’t very good at learning interesting things.”
EXPLORING ARTIFICIAL INTELLIGENCE:
With nearly half of all media and media tech companies incorporating Artificial Intelligence into their operations or product lines, AI and machine learning tools are rapidly transforming content creation, delivery and consumption. Find out what you need to know with these essential insights curated from the NAB Amplify archives:
- This Will Be Your 2032: Quantum Sensors, AI With Feeling, and Life Beyond Glass
- Learn How Data, AI and Automation Will Shape Your Future
- Where Are We With AI and ML in M&E?
- How Creativity and Data Are a Match Made in Hollywood/Heaven
- How to Process the Difference Between AI and Machine Learning
In the 1980s, a technique called backpropagation was rediscovered; and this was later combined into something that has come to be known as convolutional neural networks (CNNs). CNNs were no match for the entrenched AI methods in computer vision of the time but during the 2000s, more and more learning techniques and algorithmic improvements were added, leading to what is now known as deep learning.
“In 2012, suddenly, and seemingly out of nowhere, deep learning outperformed the standard computer vision algorithms in a set of test images of objects, known as ImageNet. The poor cousin of computer vision triumphed, and it completely changed the field of AI.”
The message is that such disruptions never stop. “Those standing tall today will be surprised by new methods that they have not begun to consider. There are small groups of renegades trying all sorts of new things, and some of them, too, are willing to labor quietly and against all odds for decades. One of those groups will someday surprise us all.”